Towards Tracardi Version 2.0: Transforming into a Fact Sourcing Platform
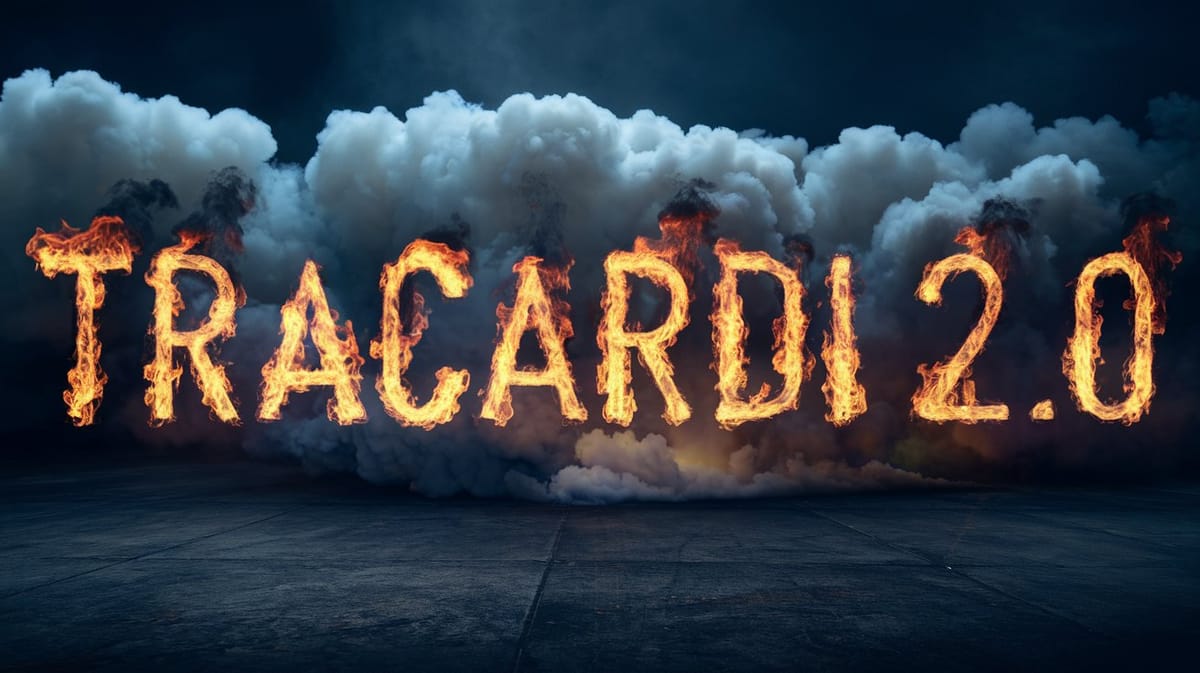
Introduction:
In the rapidly evolving landscape of data-driven decision making, organizations increasingly recognize the limitations of traditional customer data platforms (CDPs). These systems typically focus on collecting and analyzing user-centric data, often falling short when it comes to understanding the broader picture of how businesses operate. Tracardi 2.0 is designed to address this limitation. It evolves from a CDP into a powerful Fact Sourcing Platform (FSP), aiming to collect, organize, and process not just customer data but the full spectrum of business interactions and operational events.
This article presents the vision behind Tracardi 2.0, the motivations for its transformation, and the key architectural and functional advancements that distinguish it from its predecessor.
The Concept of a Fact Sourcing Platform:
At the heart of Tracardi 2.0 lies the concept of the "fact"—a structured event that encapsulates not only what happened but also who was involved, where it occurred, and under what conditions. Unlike traditional events, a fact in Tracardi is a comprehensive record that binds together multiple entities such as customers, products, transactions, and time-based context.
Facts are more than just logs; they are atomic pieces of truth that can be connected and recombined to form a larger, more meaningful view of a business ecosystem. This ability to transform raw events into interlinked facts makes Tracardi 2.0 a truly intelligent data platform.
From Event Logging to Knowledge Graphs:
Every fact in Tracardi becomes a node in a dynamic knowledge graph. As more facts are collected, the system automatically builds a network of interconnected data points that reflect the real-world relationships between business entities. For example, a delivery event can be linked to a purchase, which in turn is associated with a product and a customer. These relationships allow the platform to "understand" the implications of each fact in context.
This structure enables Tracardi to go beyond analytics and enter the domain of automation and AI. By analyzing paths and patterns within the graph, Tracardi can trigger specific actions, such as sending a follow-up email or alerting a manager, based on rule-based logic or machine-learned behavior.
Flexible Entity Identification:
One of the foundational requirements for building reliable data relationships is entity identification. Tracardi 2.0 introduces customizable identification protocols. Users can define what uniquely identifies an entity: it could be an email address, a phone number, a name, or even a combination of fields.
This flexibility allows the system to either merge related events into a single entity or to keep them separate when the identifiers differ. It also prevents erroneous merges, which is crucial for maintaining data integrity, especially in compliance-heavy environments.
Granular Historical Tracking:
Each entity in Tracardi 2.0 is associated with a full history of related facts. This historical tracking allows for real-time insights into the entity's state. For example, a product might have a timeline showing manufacturing, shipment, delivery, returns, and customer feedback. Similarly, a customer profile could reflect not just purchases but support interactions, browsing behavior, and marketing engagement.
By maintaining this deep history, Tracardi can accurately depict the lifecycle of any entity in the system, enabling better reporting, prediction, and intervention.
From Elasticsearch to Data Warehouse:
A significant architectural change in Tracardi 2.0 is the move away from Elasticsearch towards a relational Data Warehouse. This decision stems from the need to handle more complex data relationships and to support advanced analytical workloads.
The Data Warehouse not only stores facts and entity relationships but also supports efficient querying, aggregations, and time-series analysis. It becomes the foundation for business intelligence and machine learning pipelines.
Multi-Industry Use Cases:
Tracardi’s new architecture opens it up to a variety of industries. In manufacturing, facts might include machine status updates, maintenance logs, and quality assurance results. In healthcare, they could encompass patient visits, diagnostic tests, and prescription history. In finance, facts might track transactions, approvals, fraud alerts, and regulatory checks.
Tracardi 2.0 allows companies to see not only what has happened but why—and what is likely to happen next. It becomes a tool not just for marketing and customer support but for operations, compliance, and strategy.
Inference and Fact-Based Automation:
One of the most powerful features of Tracardi 2.0 is its ability to infer new facts from existing ones. For example, if two entities frequently appear together in related facts, the system can infer a relationship—such as a customer and a support agent, or two collaborating employees.
These inferred relationships feed into the knowledge graph and can power automations. For example, if a delivery is late and the same customer has raised complaints before, the system can preemptively offer compensation or notify a supervisor. Automation is no longer based on static triggers, but on dynamic patterns.
Conclusion:
Tracardi Version 2.0 marks a pivotal shift from customer-focused data collection to comprehensive, business-wide fact sourcing. By integrating entity tracking, customizable identification, a relational data warehouse, and a powerful knowledge graph structure, Tracardi becomes more than a platform—it becomes a strategic asset for data-driven organizations.
This transformation empowers businesses to act not only on what they know, but on what their systems can intelligently deduce—making Tracardi a key player in the future of smart enterprise automation.