Navigating the Present and Future of Autonomous AI Tasks
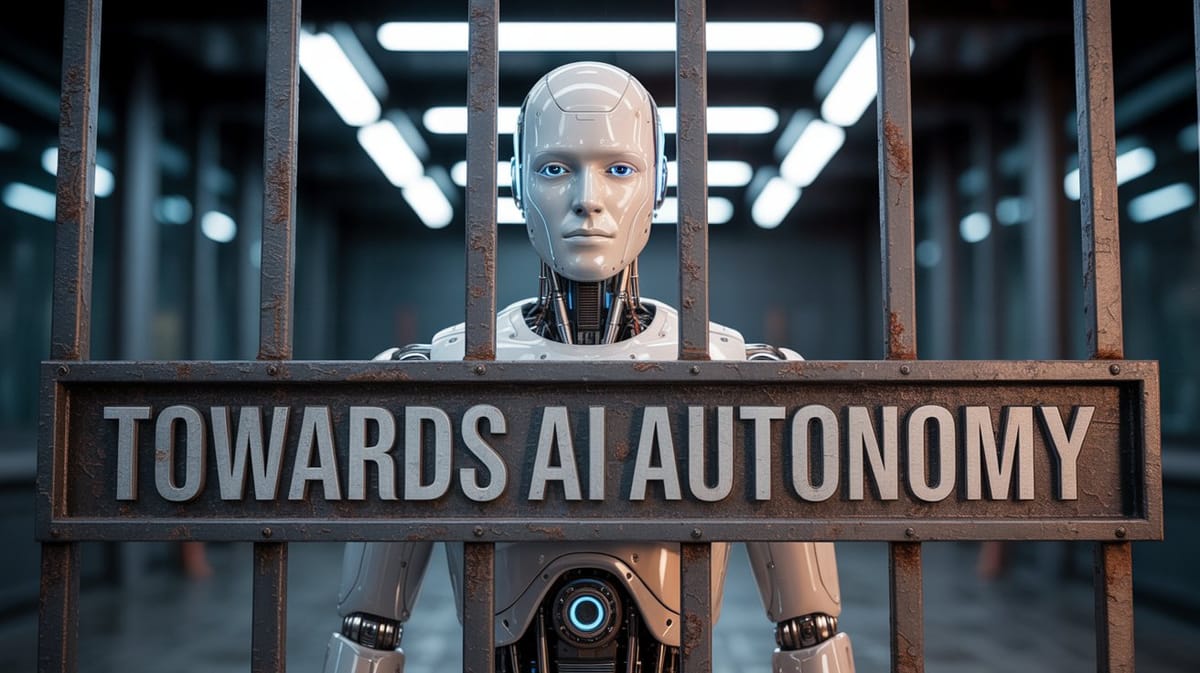
Hi there! Today, we’re diving into how artificial intelligence is currently used in autonomous tasks. While AI has tremendous potential, it still often requires human supervision. Let’s explore three scenarios where AI can operate autonomously with the right constraints and data.
Understanding Autonomous AI: The Balance of Power and Precision
Before we jump into the specific use cases, it’s essential to define what we mean by "autonomous AI." In this context, autonomy refers to an AI system's ability to perform tasks without direct human intervention. This does not imply full independence in the way a human operates but rather the ability to complete a well-defined task when operating within a specific framework of rules and data.
AI has long since surpassed the capability of mere data processing. With advancements in natural language processing, pattern recognition, and predictive analytics, AI has become a powerful engine driving insights, automation, and innovation across industries. Yet, true autonomy in AI remains elusive unless we craft clear operational boundaries, ensure consistent data quality, and create frameworks for interpretability and error handling.
Let’s delve into three scenarios that showcase how AI achieves limited yet effective autonomy today.
Example 1: Automated Content Generation
One of the most transformative uses of AI is in automated content generation. Whether it’s e-commerce product descriptions, SEO-focused blog entries, or social media content, AI models—especially those trained on large language datasets—can generate high-quality, readable content autonomously.
Imagine an e-commerce platform with thousands of new products being added weekly. Hiring content writers to produce descriptions for each item would be costly and time-consuming. Instead, an AI model can pull structured data—like specifications, brand, category, and price—and automatically craft a coherent and engaging product description.
Furthermore, AI can suggest related products by analyzing purchasing trends, user preferences, and metadata tags. For instance, if a user is viewing a smartphone, the AI might recommend cases, wireless chargers, and screen protectors. This cross-referencing capability requires no human input once properly configured.
The key here is the scope and constraints: the AI is not writing open-ended content or speculating. It’s working within a defined structure, with a clear input-output relationship. When the input data is high quality and the parameters are tightly managed, the AI system can be trusted to operate with autonomy.
Content Generation Beyond Products
AI’s capabilities extend beyond e-commerce. News organizations use AI to generate data-heavy reports like financial summaries and sports recaps. With access to statistics and predefined templates, the AI can quickly create grammatically sound, factual content. In such domains, human writers are freed up to work on more creative or investigative tasks.
Even in academia, AI tools are being used to draft literature reviews or synthesize research summaries, though these applications still require human validation due to the higher complexity and nuance involved.
Example 2: Structuring Unstructured Data
A second powerful domain of autonomous AI is structuring unstructured data. Think about how much of the world’s data is unstructured—emails, chat logs, browsing history, social media posts, and more. AI tools today can analyze this data to extract actionable insights.
Take user clickstream data as an example. Every click, hover, and scroll on a website is a piece of a larger behavioral puzzle. By applying AI models—especially those using time-series analysis and clustering algorithms—companies can identify user interests, segment customer profiles, and detect anomalies or shifts in behavior.
For instance, if an AI system detects that a user repeatedly searches for hiking gear, views related blog posts, and adds boots to a wishlist, it might autonomously conclude that the user has a growing interest in outdoor activities. With this profile established, AI can recommend content or products that align with the user’s interest, all without direct human analysis.
The autonomy here stems from the system’s ability to access structured sources (event logs, databases) and predefined models (behavior trees, customer segmentation templates). The challenge lies in ensuring that the system doesn't overstep or misinterpret noise as signal. Thus, while the processing is autonomous, the design of the system’s logic must still be human-curated.
Handling Data Variability and Ambiguity
A common concern with unstructured data is variability. Human language is full of ambiguities, sarcasm, and cultural nuances. AI systems must be trained to handle such variability—often through large-scale pretraining and fine-tuning on domain-specific corpora.
Still, when the domain is tightly controlled (e.g., customer service chats or in-app behavior), AI can operate reliably. It can categorize feedback into positive or negative sentiment, detect frequently mentioned issues, or even highlight potential improvements in product design based on recurring complaints.
Example 3: Combining Insights for Engagement
The real magic happens when we combine content generation and data structuring to enhance user engagement. This represents a higher level of autonomous AI—an orchestration of various autonomous subsystems to deliver end-to-end experiences.
Let’s say the AI system identifies a user interested in DSLR cameras based on past browsing and click patterns. It can then:
- Automatically generate a tailored product recommendation email.
- Include AI-written content about "Top Tips for Choosing Your First DSLR."
- Suggest accessories that match the specific model the user viewed.
- Provide a subscription option for similar content or promotional updates.
All of this can happen autonomously, assuming the system has access to structured product data, a content generation model, and a behavioral insights engine. With proper integration and well-defined workflows, the AI acts as a cohesive recommendation and communication engine.
Personalization Without Oversight
What’s powerful here is the personalization aspect. Traditional marketing campaigns often cast a wide net. AI-driven systems can operate at a 1:1 personalization scale, tailoring each interaction to an individual user. This level of nuance is incredibly valuable for customer retention, satisfaction, and conversion.
Because the AI is drawing from structured inputs (user behavior, product databases) and confined to predefined communication strategies, human oversight isn’t necessary on a day-to-day basis. Teams only need to intervene when making strategic adjustments, retraining models, or expanding into new domains.
The Risks and Responsible Design of Autonomous AI
While the benefits are clear, there are risks that come with AI autonomy. Bias in data can lead to flawed outputs. Over-personalization might feel invasive. Misinterpretation of ambiguous behavior can result in irrelevant suggestions. To manage these risks, we must design AI systems with clear boundaries, fallback mechanisms, and transparency.
For example, if an AI model generates content based on user feedback, it must be vetted for compliance and appropriateness. Similarly, systems structuring behavioral data should anonymize inputs to protect user privacy.
In regulated industries—like finance or healthcare—the bar is even higher. AI autonomy must be paired with explainability. Every autonomous decision should be auditable and justified in terms of the input data and model logic.
Conclusion: The Path Forward for Autonomous AI
In summary, while AI still needs some human oversight, these examples show that with the right constraints and well-defined data, AI can handle many tasks autonomously. Automated content generation, unstructured data processing, and engagement orchestration are just the beginning.
The future of AI lies in balancing its vast knowledge with well-managed limitations. The goal is not to eliminate human involvement entirely but to shift it toward design, strategy, and oversight. With responsible design, AI can free humans from repetitive tasks and elevate our capacity for innovation and creativity.
We are only scratching the surface of what’s possible. As AI models grow more sophisticated and datasets more refined, the scope for autonomous operations will expand. But the core principle remains: autonomy thrives not in chaos, but in clarity.
Stay curious, and keep exploring the edges of human-machine collaboration!